The New King of Tech
How Jensen Huang built Nvidia into a nearly $3 trillion business
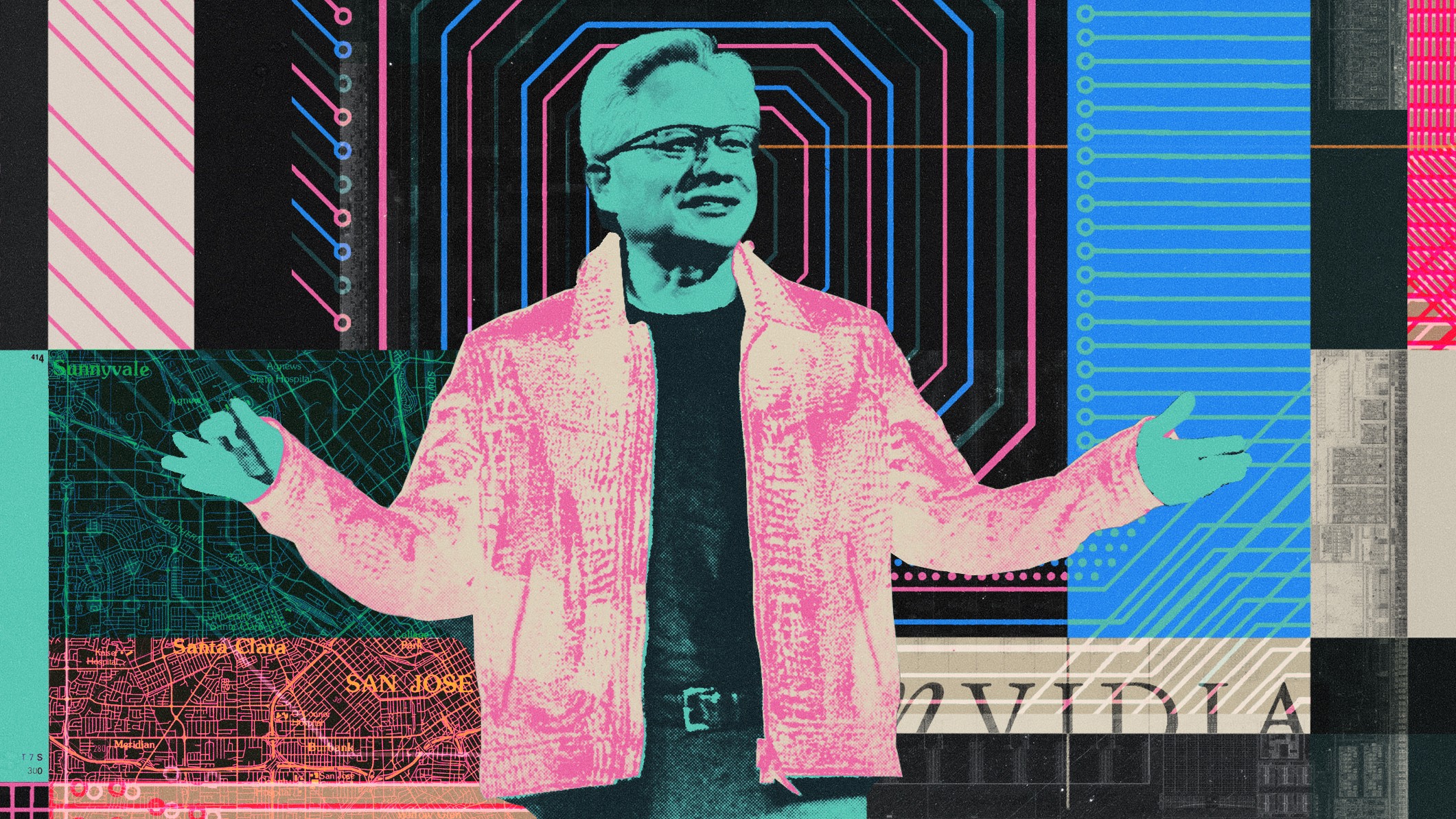
Another day, another new AI large language model that’s supposedly better than all previous ones. When I began writing this story, Elon Musk’s xAI had just released Grok 3, which the company says performs better than its competitors against a wide range of benchmarks. As I was revising the article, Anthropic released Claude 3.7 Sonnet, which it says outperforms Grok 3. And by the time you read this, who knows? Maybe an entirely new LLM will have appeared. In January, after all, the AI world was temporarily rocked by the release of a low-cost, high-performance LLM from China called DeepSeek-R1. A month later, people were already wondering when DeepSeek-R2 would come out.
The competition among LLMs may be hard to keep track of, but for Nvidia, the company that designs the computer chips—or graphics-processing units (GPUs)—that many of these large language models have been trained on, it’s also enormously lucrative. Nvidia, which, as of this writing, is the third-most-valuable company in the world (after Apple and Microsoft), was started three decades ago by engineers who wanted to make graphics cards for gamers. How it evolved into the company that is providing almost all the picks and shovels for the AI gold rush is the story at the core of Stephen Witt’s The Thinking Machine. Framed as a biography of Jensen Huang, the only CEO Nvidia has ever had, the book is also something more interesting and revealing: a window onto the intellectual, cultural, and economic ecosystem that has led to the emergence of superpowerful AI.
[James Surowiecki: DeepSeek’s chatbot has an important message]
That ecosystem’s center, of course, is Silicon Valley, where Huang has spent most of his adult life. He was born in Taiwan, the son of a chemical engineer and a teacher. The family moved to Thailand when he was 5, and a few years later, his parents sent him and his older brother to the United States to escape political unrest. Eventually, his parents relocated to the U.S. as well, and Huang grew up in the suburbs of Portland, Oregon. In the early 1980s, after majoring in electrical engineering at Oregon State (which at the time didn’t offer a computer-science major), he got a job at Advanced Micro Devices. The company—then the poor cousin of the chip giant Intel—was headquartered in Sunnyvale, California, near US 101, the highway that runs from San Jose to Stanford. Since then, Huang’s career has unfolded within a five-mile radius of that office.
Huang soon left AMD for a firm called LSI Logic Corporation, which built software-design tools for chip architects, and then left LSI in 1993 to start Nvidia with the chip designers Curtis Priem and Chris Malachowsky: He was right on target “to run something by the age of thirty,” as he’d told them he aimed to do. The company was entering a crowded marketplace for developing graphics cards, the computer hardware that’s used to render images and videos. Nvidia didn’t have a real business plan, but Huang’s boss at LSI recommended him to Sequoia Capital. One of the Valley’s most important venture-capital firms, Sequoia helped the company get off the ground.
The graphics-card business was built on a perpetual upgrade cycle that forced developers into a never-ending game of performance improvement: A company was only as good as its last card. At various points in those early years, Nvidia was one misstep away from bankruptcy, and its unofficial motto became “Our company is thirty days from going out of business.”
One gets the impression that Huang liked it that way. He says his heart rate goes down under pressure, and to call him a relentless worker is to understate matters. “I should make sure that I’m sufficiently exhausted from working that no one can keep me up at night,” he once said. His reading diet features business books (which he devours). He has no obvious politics (or at least never discusses them). He’s not a gaudy philanthropist. Though devoted to his family, he’s also honest: “Lori,” he says of his wife, “did ninety percent of the parenting” of their two children. For the past 30 years, his life has clearly revolved around Nvidia.
Huang’s reluctance to talk about himself makes him a challenging subject for Witt to bring to life. But Nvidia’s employees, who almost all refer to Huang by his first name, are effusive. They “worship him—I believe they would follow him out of the window of a skyscraper if he saw a market opportunity there,” Witt writes. He later adds that they see Huang “not just as a leader but as a prophet. Jensen was a prophet who made predictions about things. And then those things came true.” He has a ferocious temper—referred to in the company as “the Wrath of Huang”—and is notorious for publicly reprimanding, at length, workers who have made mistakes or failed to deliver. But he rarely fires people and, in fact, inspires intense devotion. One of his key subordinates says, “I’ve been afraid of Jensen sometimes. But I also know that he loves me.”
[Read: Jensen Huang is tech’s new alpha dog]
Huang’s greatest strength as a CEO has been his willingness to make big, risky bets when opportunities present themselves. The first of those came when he changed the architecture of Nvidia’s chips from serial processing to parallel processing. Witt calls this move “a radical gamble,” because up to that point, no company had been able to make selling parallel-processing chips a viable business.
Serial computing is the way your computer’s central processing unit works: It executes one instruction at a time, very, very fast. Witt likens it to telling one delivery van to drop off packages in sequence. By contrast, “Nvidia’s parallel GPU acts more like a fleet of motorcycles spreading out across a city,” with the drivers delivering each package at roughly the same time. The coding required to make parallel processing work was much more complex, but if you could do it, you had access to enormous amounts of computing power.
Initially, all that power was used mainly to make computer games look and perform better. But then Huang took another big risk, remaking Nvidia’s GPUs so that they could also process massive data sets, of the kind scientists might use. As one Nvidia executive puts it, “You have a video game card on one side, but it has a switch on it. So you flick that switch, and turn the card over, and suddenly the card becomes a supercomputer.”
The fascinating thing about this decision was that Huang didn’t know who might want to buy a supercomputer in the guise of a graphics card, or how many such people were out there. He was just betting that if you make powerful tools available to people, they will find a use for them, and at a scale to justify the billions in investment.
That use—and it was big—turned out to be artificial intelligence, in particular neural-network technology. As Witt notes, just as parallel processing was revolutionizing computing, a similar revolution was happening in AI research—though no one at Nvidia was paying attention to it. AI had gone through a series of boom-and-bust cycles as researchers tried different techniques, all of which ultimately failed. One of those methods was neural networks, which tried to mimic the human brain and allow the AI to evolve new rules of learning on its own. When you train these networks on massive databases of images and text, they can, over time, identify patterns and become smarter. Neural networks had long been peripheral, partly because they’re black boxes (you can’t explain how the AI is learning, or why it’s doing what it’s doing), and partly because the computing power required to make a high-performance neural network operate was out of reach.
Parallel-processing GPUs changed all that. Suddenly, AI researchers, if they could write software well enough to get the most out of the chips, had access to sufficient computing power to allow neural networks to evolve at an extraordinary pace. In 2009, Geoff Hinton, one of the godfathers of AI research, told a conference of machine-learning experts to go buy Nvidia cards. And in 2012, one of Hinton’s students, Alex Krizhevsky, strung together two Nvidia GPUs and built and trained SuperVision (which he later renamed AlexNet). It was an AI model that could, for the first time, identify images with startling accuracy, largely because, in Witt’s words, “the GPU produced in half a minute what would have taken an Intel machine an hour and what would have taken biology a hundred thousand years.”
Huang did not immediately recognize the importance of what had happened. When he spoke at Nvidia’s annual GPU Technology Conference in 2013, he never mentioned neural networks, talking instead about weather modeling and computer graphics. But a few months later, after an Nvidia researcher named Bryan Catanzaro made a direct pitch to him about the importance of AI, Huang had what Witt calls a “Damascene epiphany”: He placed another big bet, essentially transforming Nvidia from a graphics company into an AI company over the course of a weekend. This bet was less risky than his earlier ones, because even though Nvidia had competitors who also built GPUs, none of them had really designed theirs to be used as supercomputers. Still, going all in was prescient—developments such as large language models had yet to take off—and is what has turned Nvidia into a nearly $3 trillion company.
[Read: The lifeblood of the AI boom]
That weekend feels as if it were the compressed culmination of Nvidia’s story, which isn’t empirically true. The 12 years that followed have been incredibly eventful, and incredibly profitable, as the company has kept improving its chips, servicing the insatiable appetite for computing power created by the emergence of LLMs, and fending off competitors (many of whom are Nvidia’s customers, now building their own chips). But the foundations for that pivot, and all that ensued, were already in place when Huang decided to act on his AI insight.
Those foundations, The Thinking Machine makes clear, were not laid by Nvidia alone. Indeed, among Witt’s key contributions is to show that Nvidia’s success can’t be understood apart from the culture and economy of Silicon Valley (and of tech more generally). Take the simple fact of free labor markets. One catalyst of the Valley’s success, as the scholar AnnaLee Saxenian has famously argued, was a freewheeling, risk-taking culture that encouraged workers to leave companies for competitors or to start their own firms. And that depended, in part, on the fact that noncompete clauses were unenforceable in California. Nvidia’s history exemplifies this: not just Huang’s mobility, but that of his early hires as well. Later, one of the company’s favorite tactics was to poach its competitors’ best engineers and coders—bad form, perhaps, but a good business tactic.
Nvidia also benefited from the research investments made by the government and universities. One of the crucial breakthroughs in unlocking the power of parallel computing, for instance, was an open-source programming language called Brook, which a gamer and Stanford graduate student named Ian Buck developed with a group of researchers in 2003, relying on a Defense Department grant. Alex Krizhevsky and his partner Ilya Sutskever (who later helped start OpenAI) were grad students at the University of Toronto when Krizhevsky devised AlexNet. The contest in which the model demonstrated its accuracy, the ImageNet challenge, was designed by a Stanford computer scientist named Fei-Fei Li. And as that lineup demonstrates (Krizhevsky and Sutskever were born in the Soviet Union, Li in China), immigration has been central to the history of not just Nvidia but AI generally.
Practical economic features of the ecosystem mattered as well. The most important was the rise of independent chip foundries: factories that serve many different companies and make chips on order. Nvidia’s partnership with Taiwan Semiconductor Manufacturing Company, the best-known of these factories, allowed it to become a dominant player by focusing on designing and writing software for its chips; Nvidia didn’t have to invest in actual production, which would have required prohibitive amounts of capital.
[From the September 2023 issue: Does Sam Altman know what he’s creating?]
Finally, Nvidia benefited from patience, and its board’s willingness to put long-term thinking ahead of short-term profits. Because of the gaming market, Nvidia was almost always a profitable company, but its stock price dropped nearly 90 percent two different times; it didn’t appreciate for a full 10 years after the dot-com bubble burst, while the company was spending billions turning its graphics cards into supercomputers. One familiar indictment of American capitalism is that it’s too short-term-focused. In the tech industry, at least, the trajectory of Nvidia (and many other companies) suggests that’s a bum rap.
To be sure, Huang himself was central to Nvidia’s success: He has run the company essentially on his own (as Witt puts it, he has had “no right-hand man or woman, no majordomo, no second-in-command”), and he’s made the bold moves. What’s more, he seems to have done so without a trace of doubt. Lots of people in the AI industry—including the people training LLMs—have raised concerns about AI’s dangers, but Huang is not one of them. For him, Witt writes, “AI is a pure force for progress.” Huang does not fret that it may eat all of our jobs, or replace artists, or go rogue and decide to wipe out humanity.
In fact, when Witt, stricken with existential anxiety about how AI will change the world, asks Huang whether some of these concerns might be worth pondering, he is subjected to one of his legendary tirades:
“Is it going to destroy jobs?” Huang asked, his voice crescendoing with anger. “Are calculators going to destroy math? That conversation is so old, and I’m so, so tired of it,” he said. “I don’t want to talk about it anymore … We make the marginal cost of things zero, generation after generation after generation, and this exact conversation happens every single time!”
You could write this off as an example of Upton Sinclair’s adage “It is difficult to get a man to understand something, when his salary depends upon his not understanding it!” But the fact that Huang talks about AI in terms of its impact on “marginal costs” shouldn’t be reduced to mere opportunism: It fits right in with the single-minded focus on performance that has driven him from Nvidia’s beginning. Witt at one point calls Huang a “visionary inventor.” The vision Huang has been in thrall to, though, seems to be less about grand future goals, and more about tools—about making the fastest, most powerful chips as efficiently as possible. “Existential risk” has no place in that vision. Huang’s unapologetic stance on AI is bracing in its way, especially in contrast with the public hand-wringing of many AI chieftains, fretting about the dangers of their LLMs while continuing to develop them. But he is in effect making the biggest, riskiest bet ever—not just for Nvidia, but for all of us. Let’s hope he’s right.
This article appears in the May 2025 print edition with the headline “The New King of Tech.”